Big data-based precision agriculture plays a significant role in crop improvement by leveraging advanced technologies and data analytics to enhance various aspects of crop production. Here are several ways in which big data is utilized for crop improvement in precision agriculture:
- Genomic Data Analysis:
-Genomic Sequencing: Big data analytics processes large genomic datasets to identify genetic markers associated with desirable traits in crops, such as resistance to diseases, tolerance to environmental stress, and improved yield.
- Marker-Assisted Breeding: Big data aids in identifying and selecting plants with specific genetic traits, accelerating the traditional breeding process.
- Crop Monitoring and Phenotyping:
- High-Resolution Imaging: Remote sensing technologies, including satellite imagery and drones, capture detailed information about crop health, growth, and stress. Big data analytics processes this imagery to monitor phenotypic characteristics and identify variations.
- Automated Phenotyping: Machine learning algorithms analyze images to automatically identify and quantify specific traits, enabling high-throughput phenotyping for large-scale crop trials.
- Precision Nutrient Management:
- Soil and Nutrient Analysis: Big data assists in analyzing soil composition and nutrient levels. This information is used to optimize fertilizer application, ensuring that crops receive the right nutrients in the right amounts at the right time.
- Disease and Pest Management:
- Early Detection: Big data analytics process data from various sources, including sensors and imaging, to detect early signs of diseases and pest infestations. This enables timely intervention to prevent or mitigate crop damage.
- Integrated Pest Management (IPM): Data-driven insights help in developing IPM strategies, which involve the targeted and judicious use of pesticides based on real-time monitoring and risk assessments.
- Climate Resilience:
- Climate Modelling: Big data is used to analyze historical weather patterns and predict future climate trends. This information helps farmers make informed decisions about crop selection and management practices that are more resilient to changing climate conditions.
- Data-Driven Decision Support Systems:
- Crop Modelling: Advanced models use big data to simulate and predict crop growth under different conditions. This aids farmers in making decisions related to planting times, irrigation schedules, and crop rotations.
- Prescriptive Analytics: Big data platforms provide prescriptive insights, recommending specific actions to optimize crop performance based on real-time and historical data.
- Digital Twins for Crops:
- Simulation and Modelling: Digital twins, virtual representations of crops, allow for simulation and modelling of crop behaviour under various conditions. Big data helps refine these models for more accurate predictions and insights.
- Varietal Selection:
- Performance Analytics: Data analytics enable the evaluation of crop varieties under different environmental conditions, helping farmers select the most suitable varieties for their specific locations.
- Collaborative Research and Knowledge Sharing:
- Data Sharing Platforms: Big data facilitates collaborative research by providing platforms where researchers and farmers can share data, insights, and best practices for crop improvement.
By leveraging big data in these ways, precision agriculture contributes to the continuous improvement of crop varieties, increased agricultural productivity, and the development of more sustainable and resilient farming practices.
Source: Bhat, S. A., & Huang, N. F. (2021). Big data and ai revolution in precision agriculture: Survey and challenges. IEEE Access, 9, 110209-110222.
Dr. Md. Monirul Islam
Senior Scientist
ASRBC, ACI Seed
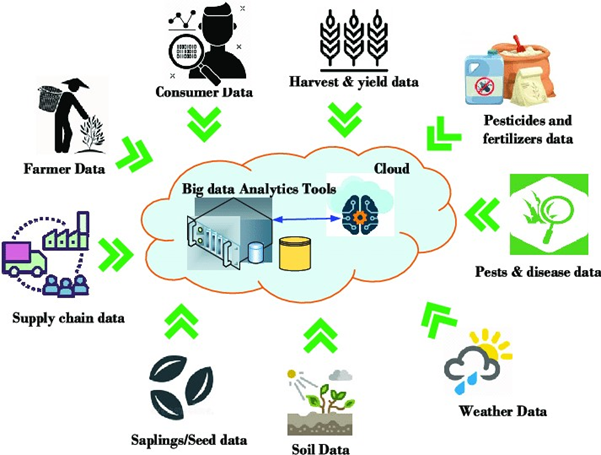